Publikationen
Ausgewählte Publikationen
Hier finden Sie ausgewählte Publikationen aus den letzten Jahren. Eine ausführliche Liste der Publikationen finden Sie auf der Google Scholar oder DBLP Seite von Stefan Schneegaß.
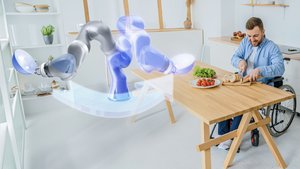
Art der Publikation: Dissertation
An Interaction Design for AI-enhanced Assistive Human-Robot Collaboration
- Autor(en):
- Pascher, Max
- Veröffentlichung:
- 2024
- ISBN:
- 978-3-00-079648-7
- Digital Object Identifier (DOI):
- doi:10.17185/duepublico/82229
- Link zum Volltext:
- https://duepublico2.uni-due.de/receive/duepublico_mods_00082229
- Zitation:
- Download BibTeX
Kurzfassung
The global population of individuals with motor impairments faces substantial challenges, including reduced mobility, social exclusion, and increased caregiver dependency. While advances in assistive technologies can augment human capabilities, independence, and overall well-being by alleviating caregiver fatigue and care receiver weariness, target user involvement regarding their needs and lived experiences in the ideation, development, and evaluation process is often neglected. Further, current interaction design concepts often prove unsatisfactory, posing challenges to user autonomy and system usability, hence resulting in additional stress for end users. Here, the advantages of Artificial Intelligence (AI) can enhance accessibility of assistive technology. As such, a notable research gap exists in the development and evaluation of interaction design concepts for AI-enhanced assistive robotics.
This thesis addresses the gap by streamlining the development and evaluation of shared control approaches while enhancing user integration through three key contributions. Firstly, it identifies user needs for assistive technologies and explores concepts related to robot motion intent communication. Secondly, it introduces the innovative shared control approach Adaptive DoF Mapping Control (ADMC), which generates mappings of a robot’s Degrees-of-Freedom (DoFs) based on situational Human-Robot Interaction (HRI) tasks and suggests them to users. Thirdly, it presents and evaluates the Extended Reality (XR) framework AdaptiX for in-silico development and evaluation of multi-modal interaction designs and feedback methods for shared control applications.
In contrast to existing goal-oriented shared control approaches, my work highlights the development of a novel concept that does not rely on computing trajectories for known movement goals. Instead of pre-determined goals, ADMC utilises its inherent rule engine – for example, a Convolutional Neural Network (CNN), the robot arm’s posture, and a colour-and-depth camera feed of the robot’s gripper surroundings. This approach facilitates a more flexible and situationally aware shared control system.
The evaluations within this thesis demonstrate that the ADMC approach significantly reduces task completion time, average number of necessary switches between DoF mappings, and perceived workload of users, compared to a non-adaptive input method utilising cardinal DoFs. Further, the effectiveness of AdaptiX for evaluations in-silico as well as real-world scenarios has been shown in one remote and two laboratory user studies.
The thesis emphasises the transformative impact of assistive technologies for individuals with motor impairments, stressing the importance of user-centred design and legible AI-enhanced shared control applications, as well as the benefits of in-silico testing. Further, it also outlines future research opportunities with a focus on refining communication methods, extending the application of approaches like ADMC, and enhancing tools like AdaptiX to accommodate diverse tasks and scenarios. Addressing these challenges can further advance AI-enhanced assistive robotics, promoting the full inclusion of individuals with physical impairments in social and professional spheres.